소비자가 AI 서비스를 사용할 때 다 좋아하는 것은 아니다, 만족도가 천차만별이라고 하셨어요. 어떤 의미인가요?
“데이트 충고처럼 취향이 관련되거나 음식 추천처럼 감각이 동반되는 경우, 사람들은 AI 서비스를 받는 것을 불편해합니다. 또한 최종 의사결정이 AI에 의해서 내려지는 것도 받아들이지 못합니다.”
*행동경제학개론
기업의 AI 활용법
– 기업의 #AI 활용 시 소비자 행동 분석
– AI의 의인화가 가져오는 역효과
– AI 서비스의 만족도와 활용법
– #주재우 교수 (국민대 경영학과)
#kbs1라디오 #라디오 #KBS라디오 #시사라디오 #KBS1Radio #성공예감이대호입니다 #성공예감 #이대호 #경제 #투자
***
Reference 1
Longoni, C., & Cian, L. (2022). Artificial intelligence in utilitarian vs. hedonic contexts: The “word-of-machine” effect. Journal of Marketing, 86(1), 91–108.
Rapid development and adoption of AI, machine learning, and natural language processing applications challenge managers and policy makers to harness these transformative technologies. In this context, the authors provide evidence of a novel “word-of-machine” effect, the phenomenon by which utilitarian/hedonic attribute trade-offs determine preference for, or resistance to, AI-based recommendations compared with traditional word of mouth, or human-based recommendations. The word-of-machine effect stems from a lay belief that AI recommenders are more competent than human recommenders in the utilitarian realm and less competent than human recommenders in the hedonic realm. As a consequence, importance or salience of utilitarian attributes determine preference for AI recommenders over human ones, and importance or salience of hedonic attributes determine resistance to AI recommenders over human ones (Studies 1–4). The word-of machine effect is robust to attribute complexity, number of options considered, and transaction costs. The word-of-machine effect reverses for utilitarian goals if a recommendation needs matching to a person’s unique preferences (Study 5) and is eliminated in the case of human–AI hybrid decision making (i.e., augmented rather than artificial intelligence; Study 6). An intervention based on the consider-the-opposite protocol attenuates the word-of-machine effect (Studies 7a–b).
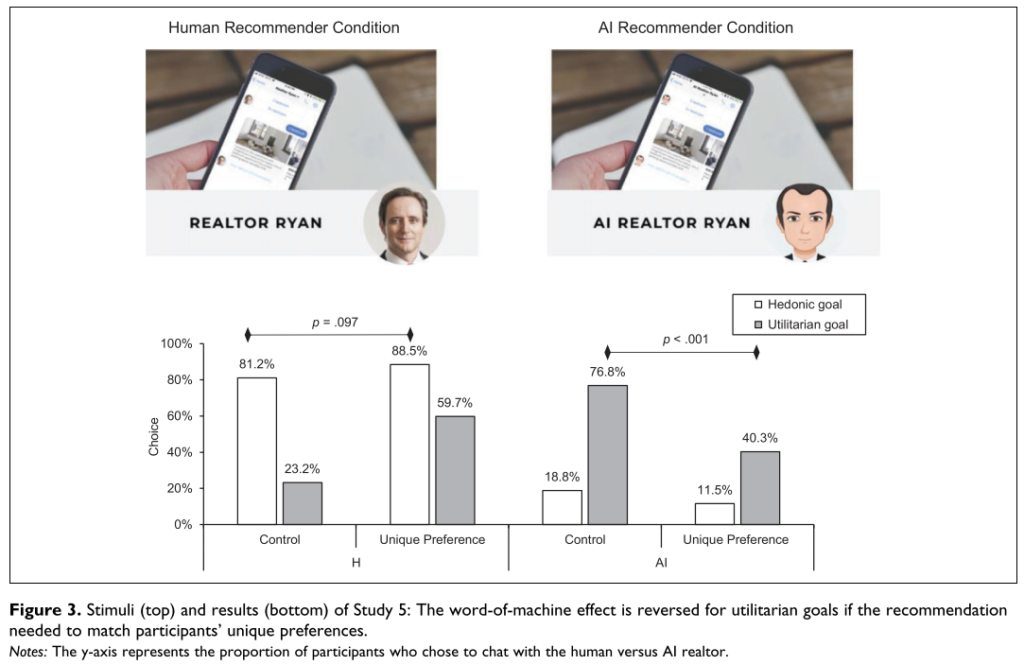
“We assessed choice on the basis of the proportion of participants who decided to chat with the human versus AI Realtor by using a logistic regression with goal, matching, and their two-way interaction as independent variables (all contrast coded) and choice (0 = human, 1 = AI) as a dependent variable. We found significant effects of goal (B = 1.75, Wald = 95.70, 1 d.f., p < .000) and matching (B = .54, Wald = 24.30, 1 d.f., p < .000). More importantly, goal interacted with matching (B = .25, Wald = 5.33, 1 d.f., p = .021). Results in the control condition (when unique preference matching was not salient) replicated prior results: in the case of an activated utilitarian goal, a greater proportion of participants chose the AI Realtor (76.8%) over the human Realtor (23.2%;z = 8.91, p < .001), and when a hedonic goal was activated, a lower proportion of participants chose the AI (18.8%) over the human Realtor (81.2%;z = 10.35, p < .001). However, making unique preference matching salient reversed the word-of-machine effect in the case of an activated utilitarian goal: choice of the AI Realtor decreased to 40.3% (from 76.8% in the control; z = 6.17, p < .001). That is, making unique preference matching salient turned preference for the AI Realtor into resistance despite the activated utilitarian goal, with most participants choosing the human over the AI Realtor. In the case of an activated hedonic goal, making unique preference matching salient further strengthened participants’ choice of the human Realtor, which increased to 88.5% from 81.2% in the control, although the effect was marginal, possibly due to a ceiling effect (z = 1.66, p = .097).
Overall, whereas the word-of-machine effect replicated in the control condition when unique preference matching was salient, participants preferred the human Realtor over the AI recommender both in the hedonic goal conditions (human = 88.5%,AI = 11.5%;z = 12.40, p < .001) and in the utilitarian goal conditions (human =59.7%,AI = 40.3%;z = 3.24, p = .001; Figure 3), corroborating the notion that people view AI as unfit to perform the task of matching a recommendation to one’s unique preferences.
These results show that preference matching is a boundary condition of the word-of-machine effect, which reversed in the case of a utilitarian goal when people had a salient goal to get recommendations matched to their unique preferences and needs. The next study tests another boundary condition.” (pp. 99-100)
***
Reference 2
Puntoni, S., Reczek, R. W., Giesler, M., & Botti, S. (2021). Consumers and artificial intelligence: An experiential perspective. Journal of Marketing, 85(1), 131–151.
Artificial intelligence (AI) helps companies offer important benefits to consumers, such as health monitoring with wearable devices, advice with recommender systems, peace of mind with smart household products, and convenience with voice-activated virtual assistants. However, although AI can be seen as a neutral tool to be evaluated on efficiency and accuracy, this approach does not consider the social and individual challenges that can occur when AI is deployed. This research aims to bridge these two perspectives: on one side, the authors acknowledge the value that embedding AI technology into products and services can provide to consumers. On the other side, the authors build on and integrate sociological and psychological scholarship to examine some of the costs consumers experience in their interactions with AI. In doing so, the authors identify four types of consumer experiences with AI: (1) data capture, (2) classification, (3) delegation, and (4) social. This approach allows the authors to discuss policy and managerial avenues to address the ways in which consumers may fail to experience value in organizations’ investments into AI and to lay out an agenda for future research.
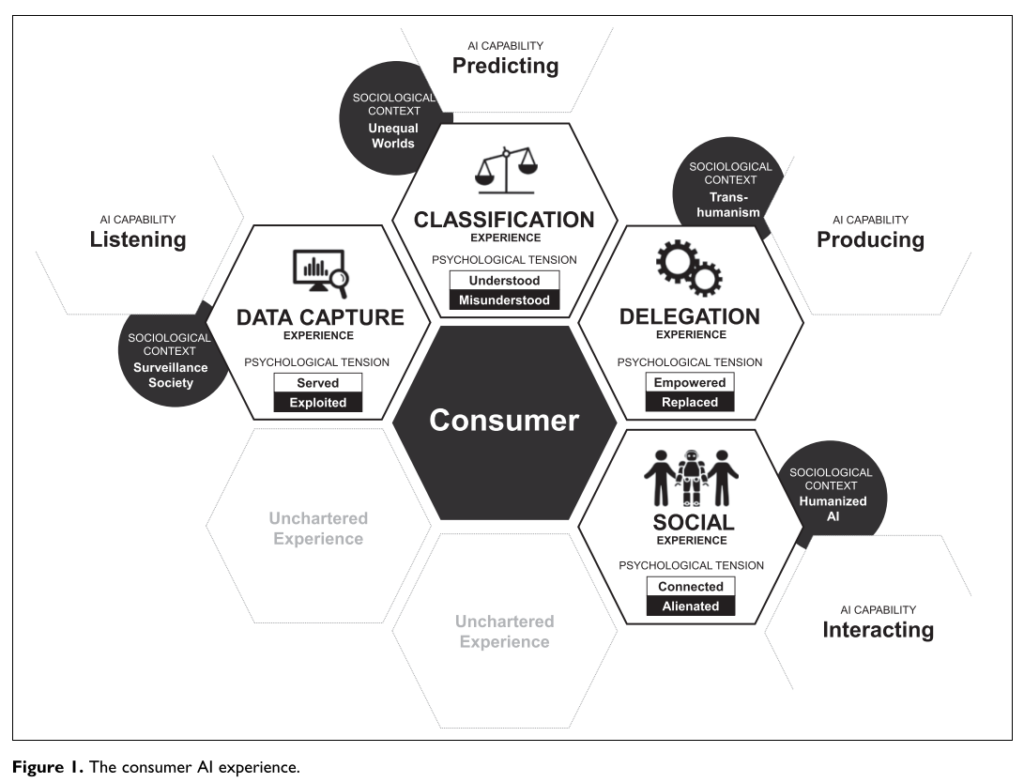
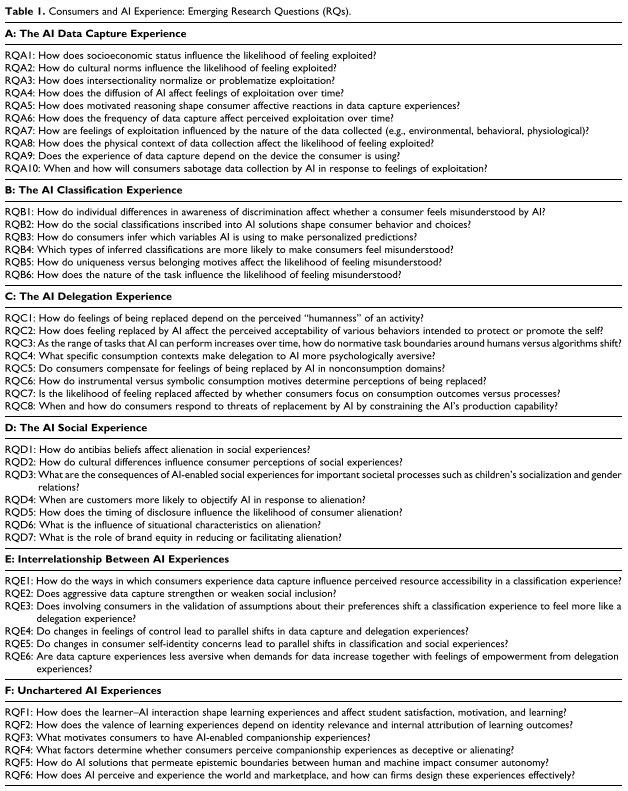